As lenders gravitate towards using artificial intelligence (AI), they must be dedicated to removing bias from their models. Luckily there are tools to help them maximize returns and minimize risks.
FairPlay.ai co-founder and CEO Kareem Saleh has been at the intersection of AI and financial inclusion for most of his career. While EVP at ZestFinance (now Zest.ai), Saleh worked with lenders to adopt AI underwriting. During the Obama administration, he oversaw $3 billion in annual investments into development-friendly projects in emerging markets.
Saleh has long studied the problem of underwriting hard-to-score borrowers, including in emerging markets like sub-Saharan Africa, Latin America, and the Caribbean, on clean energy projects and with female entrepreneurs. He was surprised to find rudimentary underwriting practices, even at the highest levels of finance.
“Not only were the underwriting methodologies extremely primitive, certainly by Silicon Valley standards, (models were built with) 20 to 50 variables, and largely in Excel,” Saleh said. “All the decisioning systems I encountered exhibited disparities toward people of color, women, and other historically underserved groups. That’s not because the people who built those models are people of bad faith. It’s largely due to limitations in data and mathematics.”
Reducing bias through fairness testing
Along with his co-founder John Merril, a Google and Microsoft veteran, Saleh believed fairness testing could be automated, providing lenders real-time visibility into how they treat different groups. He refers to FairPlay as the world’s first fairness-as-a-service company. Its client roster includes Figure, Happy Money, Splash Financial and Octane.
FairPlay allows anybody using an algorithm that makes impactful decisions to assess its fairness by answering five questions:
Is my algorithm fair?
If not, why not?
Could it be more honest?
What’s the economic impact on the business of being fairer?
Do those who get declined get a second look to see if they should have been approved?
How Capco and SolasAI reduce bias while improving risk mitigation
Capco partner Joshua Siegel helps financial services firms maximize their effectiveness. The company recently partnered with algorithmic fairness AI software provider SolasAI to reduce bias and discrimination while enhancing risk mitigation related to AI use within the financial services industry.
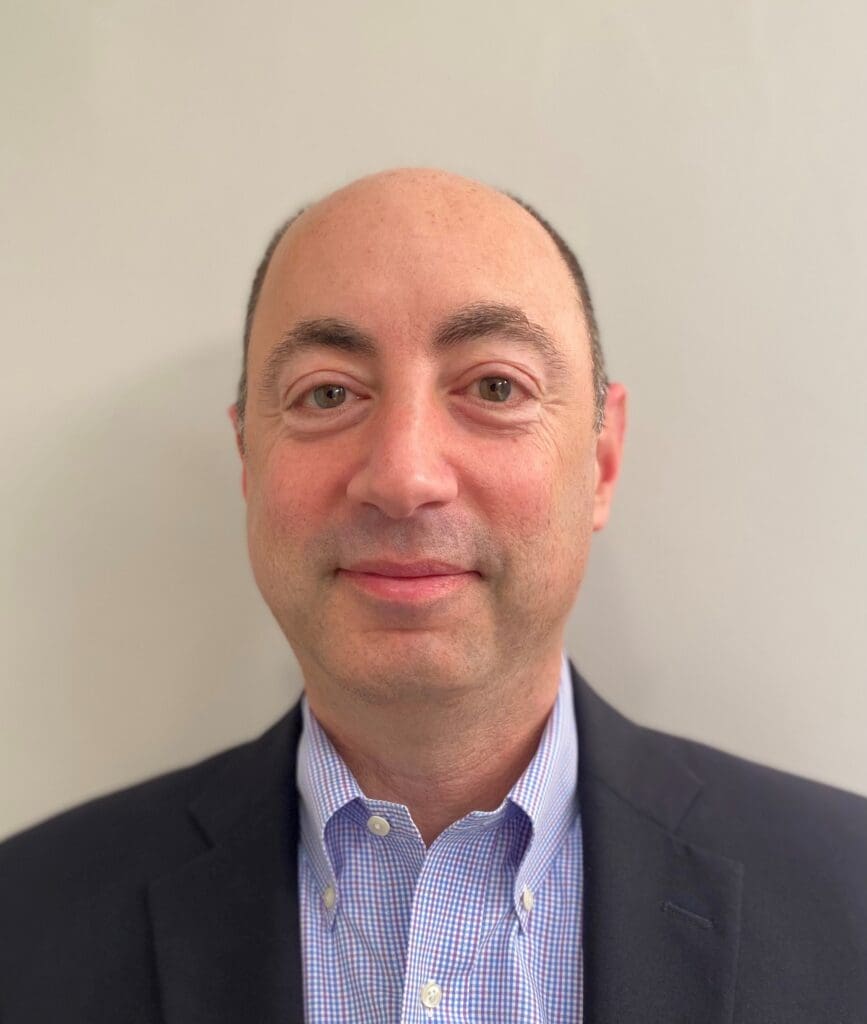
Siegel said institutions are challenged to adapt to faster innovation cycles as they seek competitive advantages. Many look to AI but need to understand the risks, which include falling short of regulatory standards.
The joint solution with SolasAI anticipates bias and quickly generates fair alternative models by integrating algorithmic fairness directly into the customer’s model-building, operations, and governance processes.??
“AI is changing the world in ways we can and cannot see,” Siegel said. “There are plenty of ways it can benefit business decisions of all types, especially lending decisions.
“While there’s much uplifting potential, there is also the risk of unintentional bias creeping into those models. And that creates reputational risk; it creates the risk of marginalizing certain communities and people institutions don’t want to marginalize.”
Also read:
Plan for scrutiny of all things AI
Organizations must expect scrutiny of anything related to AI, given media attention on AI systems’ potential for hallucinations, such as the well-publicized case where it invented court cases to support a brief. Add this to the regulatory focus on bank and fintech partnership models and their treatment of historically marginalized groups.
“…financial institutions are being asked if they take fairness seriously,” Siegel said. “They are being urged both by regulators and consumers representing the future of the financial services industry to take this more seriously and commit themselves to fixing problems when they find them.”
Police thyself to reduce bias
The problems can begin at the earliest point. Closely monitor the quality of the data used to train your models, both Saleh and Siegel cautioned. Saleh said an early model he used identified a specific small state as a prime lending territory. Upon assessment, no loans were made in what was known as a highly stringent state. Because there were no loans, the model saw no defaults and assumed the state was a goldmine.
“These things tend to error if you’re not super-vigilant about the data they consume and then the computations they’re running,” Saleh said.
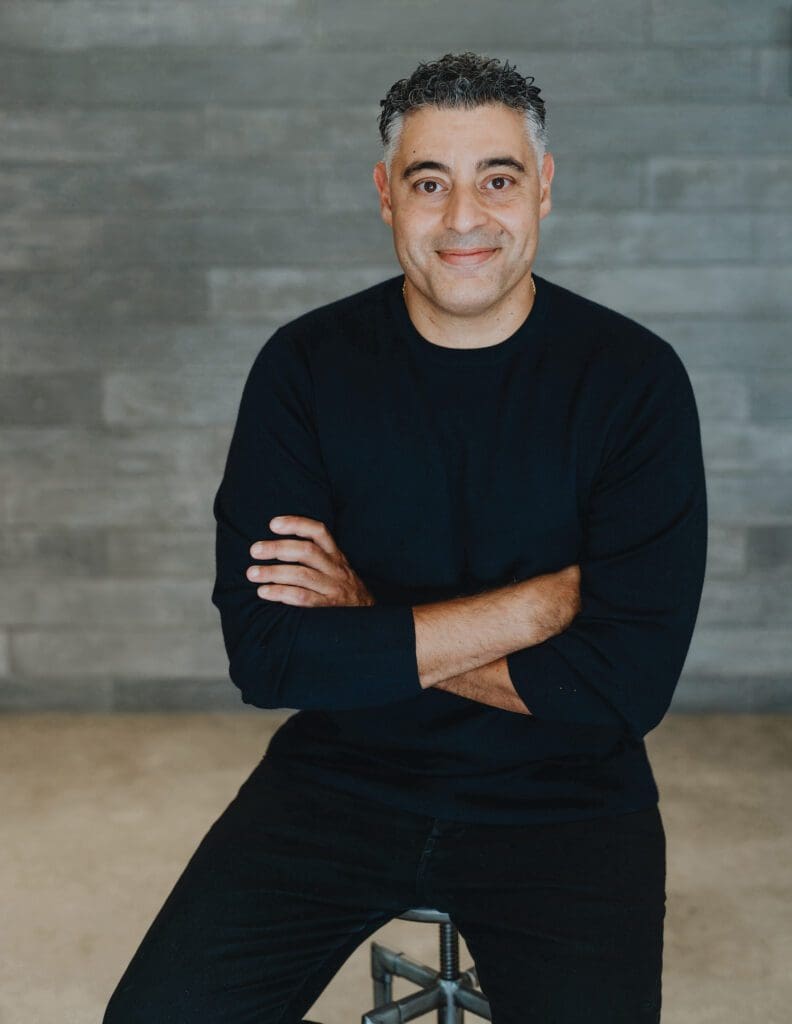
Some lenders run multiple AI systems as a check against bias. FairPlay does too. They go further by applying adversarial models that pit algorithms against each other. One predicts if another model can determine if an applicant is from a minority group. The second model asks for a decision chain to source the bias if it can.
(The first time Saleh tried the adversarial method, it showed a mortgage originator how it could increase the acceptance rate of black applicants by 10% without increasing risk.)
He added that many underwriting models strongly consider employment consistency. This hurts women between the ages of 18-45. Algorithms can be tweaked to reduce reliance on employment consistency while increasing weighting to non-prejudicial factors.
“You can still build these highly performing and predictive algorithms that also minimize biases for historically disadvantaged groups,” Saleh said. “That’s been one of the key innovations in algorithmic fairness and credit. We can do the same thing, predict who will default while minimizing disparities for protected groups.”
“That’s a way in which you can recreate the structure within the algorithm to compensate for the natural biases in the data. During the learning process, you’re forcing the model to rely on data elements that will give weight to data elements that will maximize their predictive power but minimize the disparity-driving effect.”
Be conscious of reputational risk too
Siegel’s clients want to maximize the benefit while minimizing the risk. Their solution with SolasAI identifies biases while ensuring they don’t return. The implications extend well beyond lending to marketing, human resources, and branch locations.
Institutions must guard against reputational risk, as technology makes switching to a better offer easy. If an institution is perceived as being biased in some way, it can be pilloried on social media. As recent examples show, the funds don’t take long to flood away.
“SolasAI…is a company with founders and leadership with decades of experience in fair lending and AI model construction,” Siegel said. “Their solution, which not only identifies potential variables or characteristics of a model that might be unintentionally injecting bias, (also) offers alternatives to those conditions and comes up with ways to mitigate that unintended bias while maintaining as much of the model performance as possible.
“Clients finally have the explainability and the transparency they need to benefit from AI and ensure that they’re minding the store.”
Siegel cautioned that adding conditions can weaken AI’s predictive power. Those stipulations can guide it in a specific direction instead of creating something unique.
“Rather than letting AI come to its conclusion and give it a whole set of data, it’s going to come up with correlations and causation and variables that you don’t see with your human eye,” Siegel said. “That’s a really good thing as long as you can ensure there’s nothing you didn’t want in that result.”
Possible reasons for the AI push
Is part of this push to AI motivated by lenders seeking more downstream customers compared to 15 years ago? Saleh said conventional underwriting techniques are great for scoring super-prime and prime customers where plenty of data is available. Lenders focused on those groups essentially trade customers amongst themselves.
The real growth comes from the lower-scoring groups, the thin-files, no-files, and ones with little traditional data. Since 2008, more attention has been paid to their disparate treatment, and banks don’t want to be seen as struggling to serve them.
That has driven fintech innovation as companies apply modern underwriting techniques and use unconventional data. That has enabled cashflow underwriting, which assesses data much closer to the business balance sheet.
“Cashflow underwriting is much closer to the consumer’s balance sheet than a traditional credit report,” Saleh said. “You’re taking a much more direct measure of ability and willingness to repay. The mathematics can consume lots and lots and lots of transactions to paint a finer portrait of that borrower’s ability to repay.”
How the small fish can compete with AI
Some are concerned about smaller organizations’ ability to generate sufficient data to train their AI models properly. Saleh said smaller lenders have several options, including set acquisition, bureau data, and consumer consent. The big organizations may have the data, but the smaller ones are more nimble.
“The big guys have an advantage of these amazing data repositories, although, frankly, their systems are so cobbled together in many cases, over 30 years of acquisitions, that the fact they’ve got the database does not necessarily make them fit for use,” Saleh said. “Then you’ve got the more recent entrants to the market who probably don’t have the same data as the big guys but who are much scrappier, and their data is easily put to use.
“I think everybody can play in this space.”
Prove your work
In the past, lenders could get by with only being accurate. Saleh said that now they also have to be fair, and they must be able to prove it.
There is plenty at stake. FairPlay discovered that between 25% and 33% of the highest-scoring black, brown and female declined applicants would have performed just as well as the riskiest folks most lenders approve—only a few points separate rejection from acceptance.
Saleh said the actual question facing the industry is how hard it works to find less discriminatory credit strategies. If a lender learns their model is biased, do they attempt to justify it or look for a less-biased option that also meets their business objectives?
“That’s a legal requirement in the law,” Saleh said. “It’s called the least discriminatory alternative.”
The law also makes lenders demonstrate there is no less discriminatory method for reaching those objectives. They must prove they’ve assessed their models to see if there are fairer alternatives.
And there are tools to help them do just that, tools like those offered by Capco/SolasAI and FairPlay.
“Tools like ours generate an efficient frontier of alternative strategies between perfectly fair and perfectly accurate,” Saleh said. “There are hundreds, sometimes thousands of alternative variants to a model along that spectrum. Any lender can choose what the suitable trade-off is for their business.
“I think this is a technology that very few people are using today and that everybody will be using in the not-too-distant future.”